AWS Redshift
- Amazon Redshift is a fully managed, fast, and powerful, petabyte-scale data warehouse service.
- Redshift is an OLAP data warehouse solution based on PostgreSQL.
- Redshift automatically helps
- set up, operate, and scale a data warehouse, from provisioning the infrastructure capacity.
- patches and backs up the data warehouse, storing the backups for a user-defined retention period.
- monitors the nodes and drives to help recovery from failures.
- significantly lowers the cost of a data warehouse, but also makes it easy to analyze large amounts of data very quickly
- provide fast querying capabilities over structured and semi-structured data using familiar SQL-based clients and business intelligence (BI) tools using standard ODBC and JDBC connections.
- uses replication and continuous backups to enhance availability and improve data durability and can automatically recover from node and component failures.
- scale up or down with a few clicks in the AWS Management Console or with a single API call
- distributes & parallelize queries across multiple physical resources
- supports VPC, SSL, AES-256 encryption, and Hardware Security Modules (HSMs) to protect the data in transit and at rest.
- Redshift supported only Single-AZ deployments before and the nodes are available within the same AZ, if the AZ supports Redshift clusters. However, Multi-AZ deployments are now supported for some types.
- Redshift provides monitoring using CloudWatch and metrics for compute utilization, storage utilization, and read/write traffic to the cluster are available with the ability to add user-defined custom metrics
- Redshift provides Audit logging and AWS CloudTrail integration
- Redshift can be easily enabled to a second region for disaster recovery.
Redshift Performance
- Massively Parallel Processing (MPP)
- automatically distributes data and query load across all nodes.
- makes it easy to add nodes to the data warehouse and enables fast query performance as the data warehouse grows.
- Columnar Data Storage
- organizes the data by column, as column-based systems are ideal for data warehousing and analytics, where queries often involve aggregates performed over large data sets
- columnar data is stored sequentially on the storage media, and require far fewer I/Os, greatly improving query performance
- Advance Compression
- Columnar data stores can be compressed much more than row-based data stores because similar data is stored sequentially on a disk.
- employs multiple compression techniques and can often achieve significant compression relative to traditional relational data stores.
- doesn’t require indexes or materialized views and so uses less space than traditional relational database systems.
- automatically samples the data and selects the most appropriate compression scheme, when the data is loaded into an empty table
- Query Optimizer
- Redshift query run engine incorporates a query optimizer that is MPP-aware and also takes advantage of columnar-oriented data storage.
- Result Caching
- Redshift caches the results of certain types of queries in memory on the leader node.
- When a user submits a query, Redshift checks the results cache for a valid, cached copy of the query results. If a match is found in the result cache, Redshift uses the cached results and doesn’t run the query.
- Result caching is transparent to the user.
- Complied Code
- Leader node distributes fully optimized compiled code across all of the nodes of a cluster. Compiling the query decreases the overhead associated with an interpreter and therefore increases the runtime speed, especially for complex queries.
Redshift Architecture
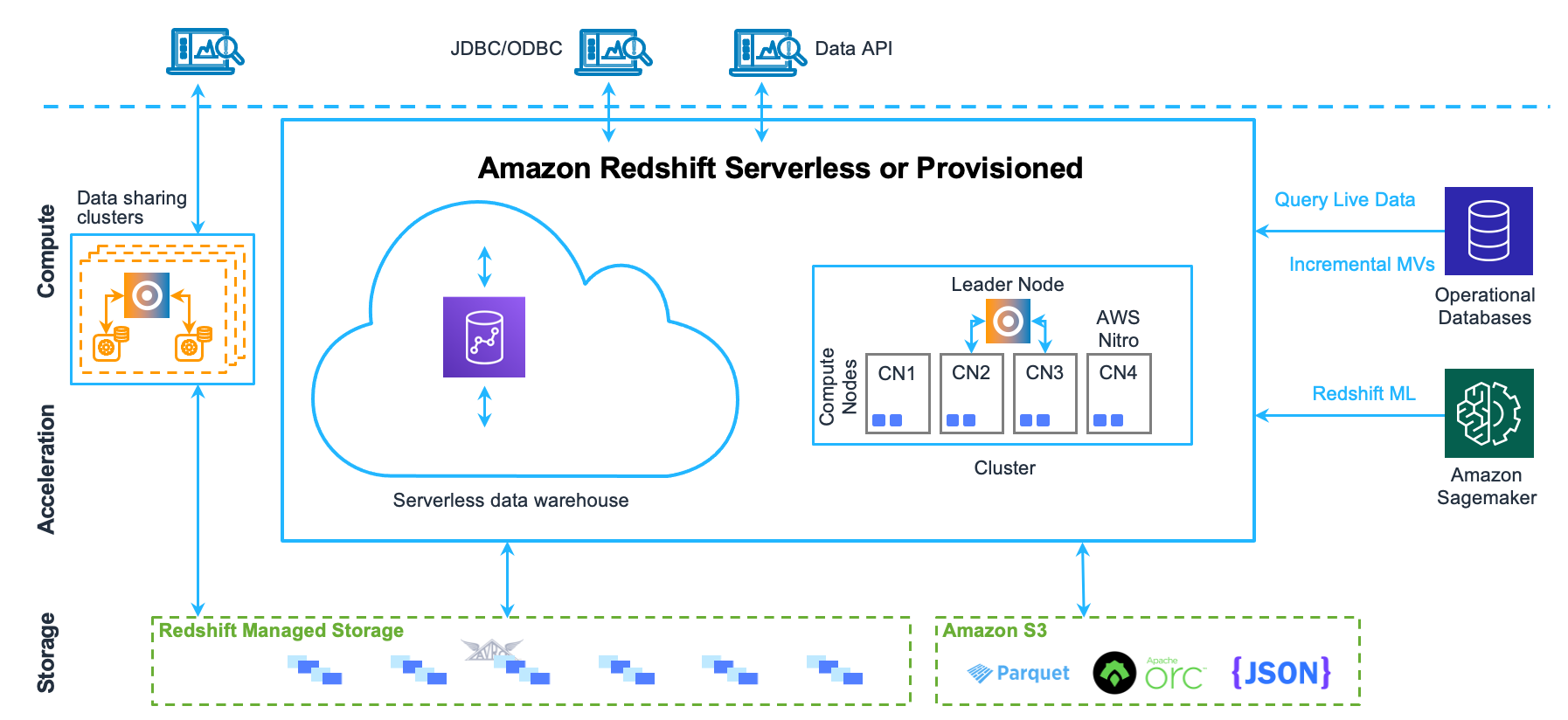
- Clusters
- Core infrastructure component of a Redshift data warehouse
- Cluster is composed of one or more compute nodes.
- If a cluster is provisioned with two or more compute nodes, an additional leader node coordinates the compute nodes and handles external communication.
- Client applications interact directly only with the leader node.
- Compute nodes are transparent to external applications.
- Leader node
- Leader node manages communications with client programs and all communication with compute nodes.
- It parses and develops execution plans to carry out database operations
- Based on the execution plan, the leader node compiles code, distributes the compiled code to the compute nodes, and assigns a portion of the data to each compute node.
- Leader node distributes SQL statements to the compute nodes only when a query references tables that are stored on the compute nodes. All other queries run exclusively on the leader node.
- Compute nodes
- Leader node compiles code for individual elements of the execution plan and assigns the code to individual compute nodes.
- Compute nodes execute the compiled code and send intermediate results back to the leader node for final aggregation.
- Each compute node has its own dedicated CPU, memory, and attached disk storage, which is determined by the node type.
- As the workload grows, the compute and storage capacity of a cluster can be increased by increasing the number of nodes, upgrading the node type, or both.
- Node slices
- A compute node is partitioned into slices.
- Each slice is allocated a portion of the node’s memory and disk space, where it processes a portion of the workload assigned to the node.
- Leader node manages distributing data to the slices and apportions the workload for any queries or other database operations to the slices. The slices then work in parallel to complete the operation.
- Number of slices per node is determined by the node size of the cluster.
- When a table is created, one column can optionally be specified as the distribution key. When the table is loaded with data, the rows are distributed to the node slices according to the distribution key that is defined for a table.
- A good distribution key enables Redshift to use parallel processing to load data and execute queries efficiently.
- Managed Storage
- Data warehouse data is stored in a separate storage tier Redshift Managed Storage (RMS).
- RMS provides the ability to scale the storage to petabytes using S3 storage.
- RMS enables scale, pay for compute and storage independently so that the cluster can be sized based only on the computing needs.
- RMS automatically uses high-performance SSD-based local storage as tier-1 cache.
- It also takes advantage of optimizations, such as data block temperature, data block age, and workload patterns to deliver high performance while scaling storage automatically to S3 when needed without requiring any action.
Redshift Serverless
- Redshift Serverless is a serverless option of Redshift that makes it more efficient to run and scale analytics in seconds without the need to set up and manage data warehouse infrastructure.
- Redshift Serverless automatically provisions and intelligently scales data warehouse capacity to deliver high performance for demanding and unpredictable workloads.
- Redshift Serverless helps any user to get insights from data by simply loading and querying data in the data warehouse.
- Redshift Serverless supports concurrency Scaling feature that can support unlimited concurrent users and concurrent queries, with consistently fast query performance.
- When concurrency scaling is enabled, Redshift automatically adds cluster capacity when the cluster experiences an increase in query queuing.
- Redshift Serverless measures data warehouse capacity in Redshift Processing Units (RPUs). RPUs are resources used to handle workloads.
- Redshift Serverless supports workgroups and namespaces to isolate workloads and manage different resources.
Redshift Single vs Multi-Node Cluster
- Single Node
- Single node configuration enables getting started quickly and cost-effectively & scale up to a multi-node configuration as the needs grow
- Multi-Node
- Multi-node configuration requires a leader node that manages client connections and receives queries, and two or more compute nodes that store data and perform queries and computations.
- Leader node
- provisioned automatically and not charged for
- receives queries from client applications, parses the queries, and develops execution plans, which are an ordered set of steps to process these queries.
- coordinates the parallel execution of these plans with the compute nodes, aggregates the intermediate results from these nodes, and finally returns the results back to the client applications.
- Compute node
- can contain from 1-128 compute nodes, depending on the node type
- executes the steps specified in the execution plans and transmits data among themselves to serve these queries.
- intermediate results are sent back to the leader node for aggregation before being sent back to the client applications.
- supports Dense Storage or Dense Compute nodes (DC) instance type
- Dense Storage (DS) allows the creation of very large data warehouses using hard disk drives (HDDs) for a very low price point
- Dense Compute (DC) allows the creation of very high-performance data warehouses using fast CPUs, large amounts of RAM and solid-state disks (SSDs)
- direct access to compute nodes is not allowed
Redshift Multi-AZ
- Redshift Multi-AZ deployment runs the data warehouse in multiple AWS AZs simultaneously and continues operating in unforeseen failure scenarios.
- Multi-AZ deployment is managed as a single data warehouse with one endpoint and does not require any application changes.
- Multi-AZ deployments support high availability requirements and reduce recovery time by guaranteeing capacity to automatically recover and are intended for customers with business-critical analytics applications that require the highest levels of availability and resiliency to AZ failures.
- Redshift Multi-AZ supports RPO = 0 meaning data is guaranteed to be current and up to date in the event of a failure. RTO is under a minute.
Redshift Availability & Durability
- Redshift replicates the data within the data warehouse cluster and continuously backs up the data to S3 (11 9’s durability).
- Redshift mirrors each drive’s data to other nodes within the cluster.
- Redshift will automatically detect and replace a failed drive or node.
- RA3 clusters and Redshift serverless are not impacted the same way since the data is stored in S3 and the local drive is just used as a data cache.
- If a drive fails,
- cluster will remain available in the event of a drive failure.
- the queries will continue with a slight latency increase while Redshift rebuilds the drive from the replica of the data on that drive which is stored on other drives within that node.
- single node clusters do not support data replication and the cluster needs to be restored from a snapshot on S3.
- In case of node failure(s), Redshift
- automatically provisions new node(s) and begins restoring data from other drives within the cluster or from S3.
- prioritizes restoring the most frequently queried data so the most frequently executed queries will become performant quickly.
- cluster will be unavailable for queries and updates until a replacement node is provisioned and added to the cluster.
- In case of Redshift cluster AZ goes down, Redshift
- cluster is unavailable until power and network access to the AZ are restored
- cluster’s data is preserved and can be used once AZ becomes available
- cluster can be restored from any existing snapshots to a new AZ within the same region
Redshift Backup & Restore
- Redshift always attempts to maintain at least three copies of the data – Original, Replica on the compute nodes, and a backup in S3.
- Redshift replicates all the data within the data warehouse cluster when it is loaded and also continuously backs up the data to S3.
- Redshift enables automated backups of the data warehouse cluster with a 1-day retention period, by default, which can be extended to max 35 days.
- Automated backups can be turned off by setting the retention period as 0.
- Redshift can also asynchronously replicate the snapshots to S3 in another region for disaster recovery.
- Redshift only backs up data that has changed.
- Restoring the backup will provision a new data warehouse cluster.
Redshift Scalability
- Redshift allows scaling of the cluster either by
- increasing the node instance type (Vertical scaling)
- increasing the number of nodes (Horizontal scaling)
- Redshift scaling changes are usually applied during the maintenance window or can be applied immediately
- Redshift scaling process
- existing cluster remains available for read operations only, while a new data warehouse cluster gets created during scaling operations
- data from the compute nodes in the existing data warehouse cluster is moved in parallel to the compute nodes in the new cluster
- when the new data warehouse cluster is ready, the existing cluster will be temporarily unavailable while the canonical name record of the existing cluster is flipped to point to the new data warehouse cluster
Redshift Security
- Redshift supports encryption at rest and in transit
- Redshift provides support for role-based access control – RBAC. Row-level access control helps assign one or more roles to a user and assign system and object permissions by role.
- Reshift supports Lambda User-defined Functions – UDFs to enable external tokenization, data masking, identification or de-identification of data by integrating with vendors like Protegrity, and protect or unprotect sensitive data based on a user’s permissions and groups, in query time.
- Redshift supports Single Sign-On SSO and integrates with other third-party corporate or other SAML-compliant identity providers.
- Redshift supports multi-factor authentication (MFA) for additional security when authenticating to the Redshift cluster.
- Redshift supports encrypting an unencrypted cluster using KMS. However, you can’t enable hardware security module (HSM) encryption by modifying the cluster. Instead, create a new, HSM-encrypted cluster and migrate your data to the new cluster.
- Redshift enhanced VPC routing forces all COPY and UNLOAD traffic between the cluster and the data repositories through the VPC.
Redshift Best Practices
Refer blog post Redshift Best Practices
Redshift vs EMR vs RDS
- RDS is ideal for
- structured data and running traditional relational databases while offloading database administration
- for online-transaction processing (OLTP) and for reporting and analysis
- Redshift is ideal for
- large volumes of structured data that needs to be persisted and queried using standard SQL and existing BI tools
- analytic and reporting workloads against very large data sets by harnessing the scale and resources of multiple nodes and using a variety of optimizations to provide improvements over RDS
- preventing reporting and analytic processing from interfering with the performance of the OLTP workload
- EMR is ideal for
- processing and transforming unstructured or semi-structured data to bring in to Amazon Redshift and
- for data sets that are relatively transitory, not stored for long-term use.
AWS Certification Exam Practice Questions
- Questions are collected from Internet and the answers are marked as per my knowledge and understanding (which might differ with yours).
- AWS services are updated everyday and both the answers and questions might be outdated soon, so research accordingly.
- AWS exam questions are not updated to keep up the pace with AWS updates, so even if the underlying feature has changed the question might not be updated
- Open to further feedback, discussion and correction.
- With which AWS services CloudHSM can be used (select 2)
- S3
- DynamoDB
- RDS
- ElastiCache
- Amazon Redshift
- You have recently joined a startup company building sensors to measure street noise and air quality in urban areas. The company has been running a pilot deployment of around 100 sensors for 3 months. Each sensor uploads 1KB of sensor data every minute to a backend hosted on AWS. During the pilot, you measured a peak of 10 IOPS on the database, and you stored an average of 3GB of sensor data per month in the database. The current deployment consists of a load-balanced auto scaled Ingestion layer using EC2 instances and a PostgreSQL RDS database with 500GB standard storage. The pilot is considered a success and your CEO has managed to get the attention or some potential investors. The business plan requires a deployment of at least 100K sensors, which needs to be supported by the backend. You also need to store sensor data for at least two years to be able to compare year over year Improvements. To secure funding, you have to make sure that the platform meets these requirements and leaves room for further scaling. Which setup will meet the requirements?
- Add an SQS queue to the ingestion layer to buffer writes to the RDS instance (RDS instance will not support data for 2 years)
- Ingest data into a DynamoDB table and move old data to a Redshift cluster (Handle 10K IOPS ingestion and store data into Redshift for analysis)
- Replace the RDS instance with a 6 node Redshift cluster with 96TB of storage (Does not handle the ingestion issue)
- Keep the current architecture but upgrade RDS storage to 3TB and 10K provisioned IOPS (RDS instance will not support data for 2 years)
- Which two AWS services provide out-of-the-box user configurable automatic backup-as-a-service and backup rotation options? Choose 2 answers
- Amazon S3
- Amazon RDS
- Amazon EBS
- Amazon Redshift
- Your department creates regular analytics reports from your company’s log files. All log data is collected in Amazon S3 and processed by daily Amazon Elastic Map Reduce (EMR) jobs that generate daily PDF reports and aggregated tables in CSV format for an Amazon Redshift data warehouse. Your CFO requests that you optimize the cost structure for this system. Which of the following alternatives will lower costs without compromising average performance of the system or data integrity for the raw data?
- Use reduced redundancy storage (RRS) for PDF and CSV data in Amazon S3. Add Spot instances to Amazon EMR jobs. Use Reserved Instances for Amazon Redshift. (Spot instances impacts performance)
- Use reduced redundancy storage (RRS) for all data in S3. Use a combination of Spot instances and Reserved Instances for Amazon EMR jobs. Use Reserved instances for Amazon Redshift (Combination of the Spot and reserved with guarantee performance and help reduce cost. Also, RRS would reduce cost and guarantee data integrity, which is different from data durability)
- Use reduced redundancy storage (RRS) for all data in Amazon S3. Add Spot Instances to Amazon EMR jobs. Use Reserved Instances for Amazon Redshift (Spot instances impacts performance)
- Use reduced redundancy storage (RRS) for PDF and CSV data in S3. Add Spot Instances to EMR jobs. Use Spot Instances for Amazon Redshift. (Spot instances impacts performance and Spot instance not available for Redshift)
References